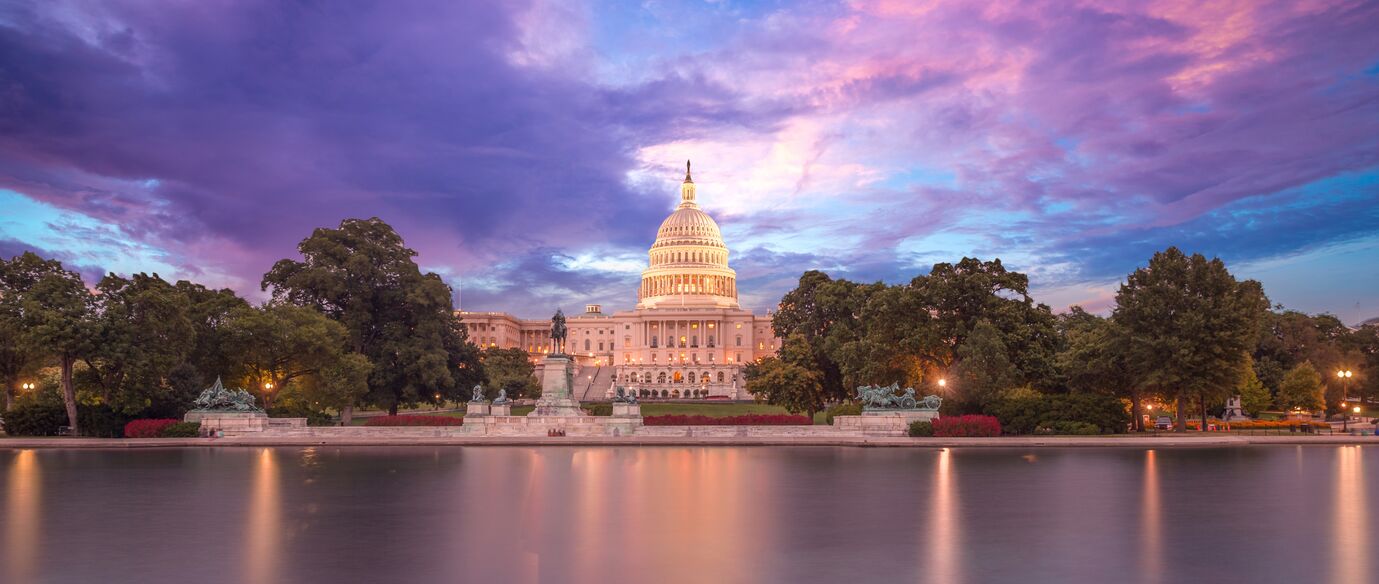
Trump Administration Executive Order (EO) Tracker
Artificial intelligence has become one of the most transformative forces in health care, reshaping everything from drug discovery to diagnostics. For pharmaceutical and medical device companies, AI-driven solutions present enormous opportunities — but they also bring new regulatory challenges. The U.S. Food and Drug Administration (FDA) is actively developing policies to keep pace with AI’s rapid evolution, and understanding this landscape is critical for industry leaders looking to innovate responsibly.
On April 29-30, Hogan Lovells and the AI Health care Coalition will host our fourth annual AI Health Law & Policy Summit. In this informative and interactive program, thought leaders and policymakers will address a variety of topics including new and emerging health care AI legislation and policy considerations, AI ethics and consumer safety, global regulatory developments, and more. The article below is the first in our thought leadership series designed to set the stage for the Summit and equip you with essential background information on this rapidly evolving landscape.
For decades, regulatory approval of medical products has followed a structured, linear process. AI, however, operates differently. Machine learning algorithms are capable of continuously refining their outputs based on new data, meaning that an AI-enabled medical device or drug development model is not necessarily static; it can evolve over time. This presents a unique challenge for regulators: how do you ensure the safety and effectiveness of a system that has the ability to always be changing?
FDA has responded by proposing new guidelines that emphasize AI based systems. Developers must provide robust validation methods that demonstrate algorithmic consistency, even as models refine themselves. Transparency is another critical factor: AI solutions must be auditable, with clear documentation detailing how they make decisions and adjust over time. Bias mitigation is also a key focus, as unbalanced training data can lead to unintended disparities in health care outcomes.
FDA has been steadily refining its approach to regulating AI in medical devices, recognizing both the immense potential and the unique challenges that AI presents in health care. Rather than treating AI like traditional software, the agency has acknowledged that AI-enabled devices require a more dynamic regulatory framework — one that accounts for continuous learning and adaptation.
In recent years, FDA has issued draft guidance outlining how developers should approach transparency, bias mitigation, and lifecycle management for AI-powered medical technologies. The agency has embraced the importance of predetermined change control plans, which allow manufacturers to update AI models while maintaining safety and effectiveness. FDA has also been proactive in approving AI-driven medical devices, with more than 1,000 AI containing products authorized through established premarket pathways. These approvals reflect the agency’s commitment to fostering innovation while ensuring that AI applications in healthcare meet rigorous safety and efficacy standards.
While FDA has not yet approved an AI powered medical device with the ability to evolve independently, technologies are moving in that direction and the agency appears to be preparing for the day that it can approve such an application. AI-enabled medical devices present another set of challenges: “algorithmic drift,” which is the tendency for AI systems to change over time as they learn from new data. To address algorithmic drift, FDA now recommends lifecycle monitoring frameworks that track AI performance long after initial approval. Companies developing AI-powered devices must implement safeguards that prevent unintended deviations from validated performance, ensuring that evolving algorithms remain trustworthy and effective.
Beyond medical devices, AI is fundamentally altering the way pharmaceuticals are developed. Drug discovery, once a slow and costly endeavor, is being accelerated by AI models capable of predicting molecular interactions with remarkable precision. AI can help predict patient outcomes, analyze disease progression, and process large datasets, but ensuring trust in AI models is crucial. Recently, FDA proposed a framework to ensure AI models used in drug and biological product submissions are credible. FDA encourages early engagement with sponsors to assess AI credibility.
FDA has released discussion papers on AI and machine learning in drug development and manufacturing. AI can assist in identifying patients who may respond better to treatments, predicting side effects, and even using digital "twins" to model medical interventions before they are applied to real patients. However, AI-driven drug development faces unique regulatory hurdles. FDA requires clear validation for AI-assisted research, ensuring that predictive models are scientifically sound. Companies must also address ethical concerns — how do we ensure that AI-generated insights do not introduce unintended biases or compromise patient safety? As AI continues to push boundaries in pharma, regulatory scrutiny will likely only intensify.
AI is reshaping clinical trials, helping researchers design studies more efficiently, recruit patients faster, and analyze data with greater precision. AI-assisted clinical trials also allow researchers to refine patient targeting, predict patient outcomes, analyze large datasets, and improve understanding of disease progression, potentially increasing trial success rates. By leveraging AI-driven algorithms, companies can streamline trial processes while improving participant diversity and accuracy.
FDA is adjusting its regulatory approach to keep up with these advancements, focusing on transparency, bias prevention, and reliable validation methods while maintaining ethical standards. AI models must be auditable and scientifically sound, ensuring they maintain integrity throughout a trial’s lifecycle. As AI takes on a bigger role in clinical research, sponsors must stay ahead of evolving FDA guidelines to ensure compliance and maintain trust.
While FDA policies govern the U.S. market, health care leaders must also consider how AI regulation varies worldwide. The European Medicines Agency (EMA) has taken a more proactive stance on AI fairness and transparency, while China’s regulators have prioritized data security concerns. Other regulators are continuing to decide their approach to regulating the use of AI and we expect there to be a range of approaches between protecting patients and encouraging innovation.
For pharmaceutical and medical device executives, strategic regulatory planning is now a necessity. AI-driven innovations must align with evolving FDA expectations, and companies should be proactive in addressing transparency, validation, and bias mitigation. Engaging with regulators early in the development process can prevent compliance pitfalls down the line.
AI regulation is a moving target, but companies that approach it thoughtfully will be better positioned to lead the next era of health care transformation. Balancing technological advancement with robust regulatory frameworks will ultimately define how AI reshapes medicine in the years ahead.
Authored by Jodi K. Scott.