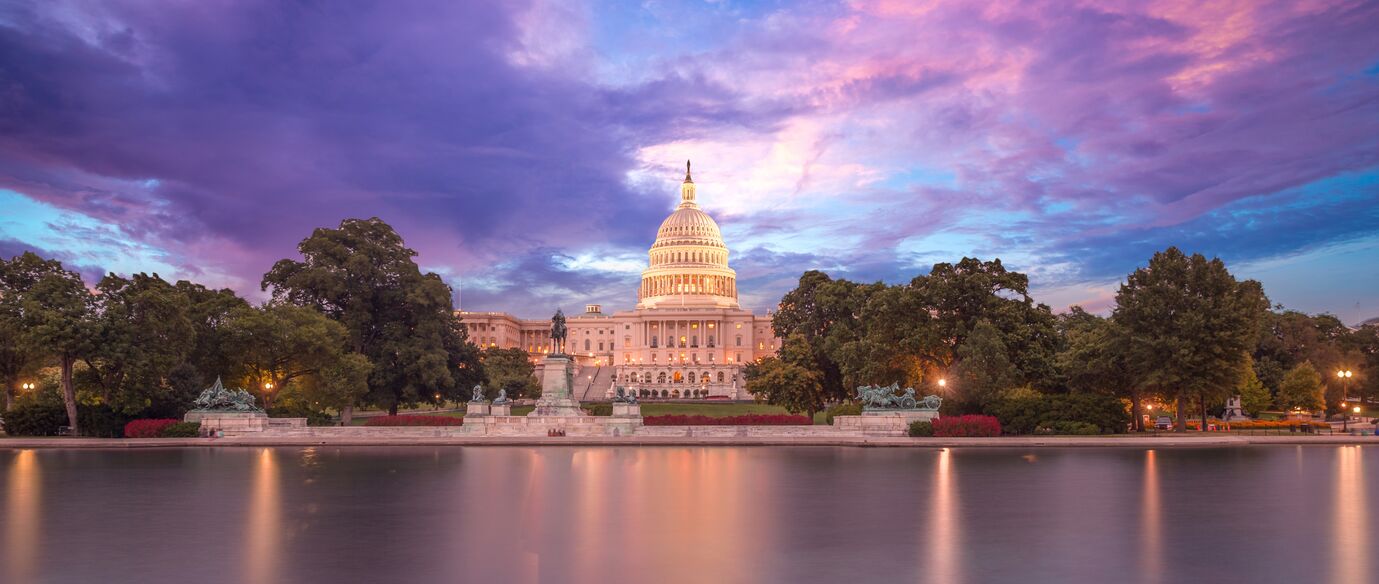
Trump Administration Executive Order (EO) Tracker
U.S. Food and Drug Administration (FDA) Commissioner Robert Califf, M.D., and other senior FDA officials recently published a “Special Communication” in JAMA describing FDA’s concerns with the use of AI in medical product development, clinical research, and clinical care, which we summarized here. We are now taking a closer look at specific issues raised by FDA in the JAMA article: (1) potential uses of AI in clinical trials, such as employing “digital twins” to generate simulated clinical records; (2) AI in medical devices; and (3) privacy considerations regarding the use of AI in clinical research.
In the JAMA article, FDA lists several areas of clinical research that could benefit from AI, including participant recruitment; selection and stratification of trial participants and sites; adherence and retention; clinical trial data collection, management, and analysis; and postmarket safety surveillance and evaluation. We do not, however, expect FDA to exercise substantial oversight over all of the AI applications developed for use in these areas. For example, using AI to match patients to studies by mining data from various sources – such as clinical trial databases, electronic health records, and social media – is not a use of AI we expect FDA to closely regulate (except to the extent that it might impact a resulting software algorithm used in a regulated product itself), since it typically does not affect the design or conduct of the clinical trial once patients have been matched to a study. Likewise, we do not expect FDA to closely regulate uses of AI that are limited to facilitating routine aspects of conducting a clinical trial, such as using AI or large language models (LLMs) for data cleaning, or to assist with drafting a clinical study report.
On the other hand, we expect FDA to exercise significant oversight over uses of AI that directly affect the design and conduct of clinical trials. An example of this kind of AI use raised by FDA in the JAMA article is the creation of “digital twins” to generate simulated clinical records to predict placebo group response. FDA previously discussed digital twins in its May 2023 discussion paper on using AI and machine learning in the development of drug and biological products, describing digital twins as “in silico representations or replicas of an individual that can dynamically reflect molecular and physiological status over time.” FDA stated that digital twins could aid clinical trial analysis by providing “a comprehensive, longitudinal, and computationally generated clinical record that describes what may have happened to that specific participant if they had received a placebo.”
Last year, FDA discussed an example where the agency provided feedback on a sponsor’s proposal to use digital twins in clinical trials of an investigational drug.1 The sponsor had proposed using digital twins to generate “patient prognostic scores” using baseline variables to predict potential placebo outcomes, thereby enabling a reduction of placebo sample sizes in the sponsor’s phase 2 and phase 3 clinical trials. FDA evaluated the sponsor’s digital twin proposal by applying a risk-based credibility assessment framework consisting of two factors:
For AI models that FDA determines are high risk, the agency may apply more stringent standards in terms of acceptance criteria and model documentation before agreeing that the AI model can be used to support a clinical trial. For the digital twins model proposed by the sponsor, FDA determined that the “model influence” was high, as there was no other factor determining the patient prognostic score. However, FDA also determined that the “decision consequence” of the digital twins model was low, as the model was expected to have minimal impact on bias and Type I error rate. Ultimately, FDA agreed that the sponsor could use the digital twins model in an exploratory analysis in its phase 2 trial, and possibly in the primary analysis in its phase 3 trial. However, FDA also requested information on whether the training dataset for the digital twins model was representative of the population the sponsor planned to enroll, and asked the sponsor to validate that the digital twins model could generate consistent patient prognostic scores for a given patient. FDA further suggested that the sponsor use explainable AI techniques (processes and methods that allow humans to better comprehend and trust AI algorithms) to identify key contributing features to the patient prognostic score.
Indeed, in new draft guidance on the use of AI to support regulatory decision-making for drug and biological products, FDA described using a risk-based approach with elements similar to those used by the agency in the digital twins example discussed above.
As FDA noted in the JAMA article, artificial intelligence and machine learning (AI/ML) have many potential uses in clinical research, including subject recruitment, trial administration including methods to gather subject clinical data, and trial surveillance. Notably, FDA may consider a particular algorithm, application, software package, or AI/ML tool to be a medical device, even when used in the clinical trial context, and therefore subject to FDA oversight. In a situation where an AI/ML software application has not received FDA market authorization, then it would be treated under FDA’s authority for investigational medical devices. Thus, understanding the current regulatory framework governing medical devices, software as a medical device (SaMD), and the use of AI/ML in medical devices is necessary to ensure compliance when utilizing these technologies in clinical trials. In fact, FDA has begun to closely scrutinize their use — including by issuing Warning Letters — as further described herein.
In general, the use of AI in a clinical trial would be considered a medical device if it satisfies the definition of a medical device found in Section 201(h) of the Federal Food, Drug, and Cosmetic Act (FDCA) and does not meet any of the statutory exclusions to that definition. Specifically, a medical device is defined as any “instrument, apparatus, implement, machine, contrivance, implant, in vitro reagent, or other similar or related article” that is:
For the avoidance of doubt, FDA has explicitly stated that AI and ML “software, when intended to treat, diagnose, cure, mitigate, or prevent disease or other conditions” will be regulated as a medical device.3 FDA typically views “screening” for a particular disease, condition, or symptom as part of the diagnostic process and is therefore inclined to regulate screening software tools as medical devices, as well.
Importantly, however, the 21st Century Cures Act narrowed the definition of a medical device in 2016 by excluding certain software functions that:
Accordingly, a detailed analysis is required to determine whether software satisfies the definition of a medical device, fails to satisfy the definition of a medical device and is therefore outside of FDA’s purview, or whether the particular use of AI may be considered the type of medical device software over which FDA intends to exercise enforcement discretion. FDA’s current views and enforcement priorities addressing SaMD, generally, are set forth in a variety of guidances (draft and final) and policy documents including:
In addition, FDA has expressed that its treatment of SaMD will largely be consistent with the risk-based approach articulated by the International Medical Device Regulators Forum (IMDRF) in the 2014 "Software as a Medical Device": Possible Framework for Risk Categorization and Corresponding Considerations”. (Though the agency’s Final Clinical Decision Support Guidance notably departed from substantial elements of the IMDRF SaMD framework that had originally been included in the draft version.)
In sum, the regulatory status of AI used in clinical trials will be determined by a multi-tier analysis, and will largely be dependent on the composition and capabilities of the software as well as its particular use in the study, with certain purposes and functions falling outside of FDA product regulation. Further, when AI/ML based software satisfies the definition of a medical device, is one for which FDA requires premarket authorization and has not received such premarket authorization, the software is subject to the requirements of 21 CFR Part 812, which governs investigational products, and may be an additional set of regulatory requirements to those which apply to the product that is the primary focus of the clinical trial. We note that even outside of the AI/ML context, FDA has questioned whether the diagnostic tools used in clinical trials have been sufficiently validated for the use they are being put to during the clinical trial – and we believe the same will hold true when the tool is an AI/ML based algorithm that may well need approval in its own right. It is also worth noting that AI/ML used in clinical trials that does not trigger regulation as a product may still be a topic of FDA inquiry, as the agency seeks to understand the impact of the use of AI on the data generated in the clinical trial.
As described above, Dr. Califf’s JAMA article captured the variety of uses for AI/ML in clinical trials. Below is an overview of potential use cases, many of which are listed in the JAMA article, with our commentary on the extent to which particular uses may satisfy the definition of a medical device and, therefore, be subject to potential FDA regulatory oversight.
Identifying Clinical Trial Sites
AI software may be used to identify optimal locations for a clinical trial site. We believe that in most cases, this function is unlikely to satisfy the definition of a medical device if it is not used in the diagnosis, treatment, mitigation, or management of disease or other conditions. This would be in line with FDA’s treatment of similar types of software applications that aid in administrative functions. However, there may be circumstances where AI-powered site selection software could potentially be considered a medical device if, by way of example, it processed patient-specific data to determine whether individual patients may possess a rare gene, issued a recommendation to test that subset of patients for the gene, and provided clinical trial site suggestions based on the results in order to enroll the targeted patients.
Selection & Recruitment of Trial Subjects
Separately, AI software used for selection and recruitment of trial subjects could potentially satisfy the definition of a medical device depending on the study design. For example, software that selects and assigns participants to a particular treatment group in a prospective study based on (i) indications that certain participants have a disease state; or (ii) a classification of the severity of participants’ disease state, could potentially satisfy the definition of a medical device because the software could be seen as assisting in the treatment or diagnosis of disease.5 By contrast, software that simply recruits participants for a study based on self-disclosed criteria, such as preexisting specific cancer diagnosis, would be less likely to satisfy the definition of a medical device if the software’s outputs could not be used to inform the diagnosis, treatment, mitigation, or management of the participant’s disease or other conditions.
It is difficult to apply a categorical rule because, as the JAMA article previewed, the possibilities of using AI for selection and recruitment are wide-ranging and may include:
Either category would largely be unregulated, but it is conceivable that that there could be situations where the application could venture into regulated territory by screening or diagnosing individual patients for purposes of evaluating eligibility for meeting inclusion/exclusion criteria.
Increasing Participant Adherence & Retention
AI software can also be implemented to increase participant adherence and retention by using smartphone reminders or alerts, e-tracking medication tools, or nonadherence alerts sent to electronic platforms. More advanced software may be capable of identifying face or voice “digital biomarkers” to remotely track adherence; or, on the extreme end, identify instances in which a patients’ condition is improving or progressing, coupled with evidence of compliance or noncompliance with treatment protocols.
Although the validation and accuracy of products that purport to detect face or voice digital biomarkers may be concerning to regulators, AI-powered software that provides participants with reminders to increase protocol adherence is less likely to require compliance with FDA regulatory requirements given that FDA’s Policy for Device Software Functions and Mobile Medical Applications provides various examples of similar products over which the agency intends to exercise enforcement discretion, including “[s]oftware functions that keep track of medications and provide user-configured reminders for improved medication adherence.”6 By contrast, AI software that utilizes a subject’s microphone to monitor a patient in a study for COPD, by listening for worsening cough symptoms over time and then issuing an alert to the patient and/or the investigator for the purpose of administering pre-specified treatment protocols, may well cross over into the realm of a regulated medical device application.
Anticipating a Trial Participant’s Clinical Outcome
It is also possible that AI could be used to anticipate a trial participant’s “clinical outcome,” which runs the risk of being considered a medical device if the information is used to inform clinical treatment decisions for trial participants. Currently, FDA treats non-AI-based software that predicts a patient’s therapeutic response as a medical device function. Thus, utilizing AI to further the same intended use would almost certainly be treated the same from a regulatory perspective, except that the agency would likely have additional concerns about how the AI was trained, tuned, and validated. We also caution that such functions may raise questions as to the scientific integrity of the study given that these sort of tools can potentially introduce bias if used too early in the study, and could similarly introduce bias if used to assign subjects to different arms of the study.
Data Cleaning & Duplicate Detection
Some AI-powered software has the potential to accomplish or assist with administrative tasks within a clinical trial such as “data cleaning” and “duplicate detection.” We believe it is unlikely AI used to accomplish administrative tasks, even within a clinical trial, would be considered a medical device function given that it would not be intended to affect the structure or function of any part of the body, and would not be intended for use in the diagnosis, cure, mitigation, treatment, or prevention of disease or other conditions. This is bolstered by the fact that administrative functions for health care facilities are exempted from the definition of a medical device by the 21st Century Cures Act. However, caution should be exercised when determining whether a particular function is purely administrative. Further, as is the case when utilizing non-AI-powered de-duplicating software, sponsors should first validate the de-duplicating function before utilizing it to de-duplicate clinical trial data due to the potential impacts on data integrity.
Discovery, Anticipatory & Predictive Use Cases
Other predictive, future-oriented, or discovery-focused functions identified by Dr. Califf and the co-authors include software designed to discover unknown symptoms or conditions, anticipate a treatment response, identify “drug candidates and outcomes,” or “predict placebo group response[s]” by generating simulated / digital twin clinical records. The determination of whether these AI software functions satisfy the definition of a medical device under FDA’s current framework would largely depend on how they were specifically used in a clinical trial, the study design, and the extent to which they were used to diagnose, treat, or prevent diseases or symptoms of participants within the trial. Still, the use of such applications to identify opportunities for product development, which are further developed and tested for a particular indication in support of an eventual marketing application, is unlikely to be viewed as a medical device.
Even if AI/ML software does not satisfy the definition of a medical device, researchers must still monitor their use of these systems because researchers and laboratories can receive FDA Warning Letters for violating their clinical trial’s investigational plan through inadequate control of artificial intelligence.7
For example, in August, 2024, FDA issued a Warning Letter to Julio R. Flamini, M.D. and the Clinical Integrative Research Center of Atlanta for failing to ensure that a study was conducted in accordance with the investigational plan because an “electronic dispensing algorithm” used in the clinical study “lacked [safeguards] to prevent errors,” resulting in a pediatric patient receiving “approximately 10 times the maximum daily dose” of a medication. In addition to Dr. Califf’s article, this Warning Letter is another data point that suggests FDA is concerned by the use of AI in clinical trials and may play a more active role to ensure current regulatory frameworks are consistently applied and enforced in this evolving space.
Thus, the importance of properly evaluating the use of AI in a clinical trial cannot be understated, given that researchers who use AI software as a medical device in a clinical trial, and fail to have their device exempted, cleared, or approved by FDA, may face serious enforcement ramifications. This could include an “It Has Come to Our Attention Letter,” which may be publicly posted on FDA’s website; a Warning Letter, which FDA publicly posts to its website and uses to identify significant violations; civil penalties; device seizures; injunctions; or, in extreme circumstances, even criminal penalties.
Several of the possible current and potential uses of AI in clinical research identified in the JAMA article have potential privacy implications and considerations. Understanding how an AI tool is used, including how the data is ingested by the tool, from where it originates, and how the data may be subsequently used, stored and/or shared through the tool, along with any legal, contractual or other limitations on the use of such data, will be critical to assessing privacy and security risks associated with the use of the tool.
As discussed above, the JAMA article mentions mining data from clinical trial databases, social media, registries and electronic health records to match potential participants to clinical trials. While the use of AI in these settings would likely be quite beneficial in terms of efficiency and improved identification of eligible participants, it also raises potential privacy risks. In particular, for data in electronic health records, the Health Insurance Portability and Accountability Act and its implementing regulations (HIPAA) could apply and impose restrictions on the use of data. For example, while HIPAA allows researchers access to protected health information (PHI) for reviews preparatory to research (including identifying potential research participants), the PHI may not leave the covered entity or be used by a third party researcher to contact potential participants without individual authorization or an IRB waiver of HIPAA authorization.
In addition, some AI tools use the data ingested to retrain models or to respond to subsequent queries using the tool. These could be prohibited under applicable privacy laws or could require additional compliance obligations. Moreover, state health privacy and sensitive condition laws also could impose limitations or requirements for the protection of patient privacy. Thus, to the extent an AI tool is used, it will be important to understand how the tool works in terms of data flows, ingestion, storage and use to confirm compliance with applicable privacy laws and the protection of personal data.
Furthermore, even web and social media scraping has been subjected to increased regulator scrutiny and litigation. New state consumer health data privacy laws in Washington and Nevada in particular require consent for the collection of consumer health data and a separate consent for sharing such information. Thus, companies and researchers may want to carefully consider the risks and ways to mitigate them before engaging in scraping or other data collection activities, as we previously discussed in greater detail online here.
The JAMA “Special Communication” further discusses the use of AI in connection with clinical trial data collection, management and analysis, including use in predicting status of and patient response to treatment, and enhancing data curation via masking and deidentification. For these uses of AI, ensuring the privacy, confidentiality and security of the data through the use of the AI tool is essential. Companies and researchers also may want to mitigate risks around the use of AI, particularly generative AI, in terms of accuracy, hallucinations, and confabulations. Although FDA acknowledges the benefits and possible uses of AI, as noted above, the agency will likely be particularly focused on the steps the researcher or company took to validate the tool and its outputs, in order to mitigate the risk that AI use could impact the quality and accuracy of the data. It will therefore be important to document these steps and the testing and validation of the AI tool, particularly where AI is used in analysis of data that will be submitted to FDA.
Finally, given the increased scrutiny around the use of AI, and the push for transparency and consent in certain circumstances where AI may be used, companies and researchers may want to consider mitigating their risk by including language in informed consent forms for studies and trials that describes their use of AI tools and software. This is particularly true where the study data will be used to train or improve an AI algorithm or model and where AI is used in a way that could impact the individual (e.g., which treatment or dosage they receive).
Medical device manufacturers, researchers, and sponsors should apply caution to prevent inadvertently implementing software that FDA regulates as a medical device. Even in cases where FDA may not necessarily regulate particular AI/ML-powered software as a medical device, the use of such software in a clinical trial may generate questions from the agency about the validity and applicability of the data. It would therefore be prudent to anticipate such questions early in the planning of any study to support FDA submissions, with special caution given to the privacy considerations raised by the use of AI in clinical trials.
As noted above, this week FDA also released new draft guidance on the use of AI to support regulatory decision-making for drugs and biological products, and for AI-enabled medical device software functions, which we will review in a forthcoming client alert. In the meantime, our team is well-acquainted with FDA’s current views of AI/ML software across a variety of applications and frequently advises on regulatory issues in this space. If you have regulatory questions surrounding the use of AI/ML in clinical trials, please contact any of the Hogan Lovells authors of this publication for assistance.
Authored by Jodi K. Scott, Robert Church, Melissa Levine, Wil Henderson, Bert Lao, Greg Kass, Lauren Massie, and Ashley Grey.
FDCA § 201(h)(1)(A-C).
FDA Discussion Paper and Request for Feedback, Proposed Regulatory Framework for Modifications to Artificial Intelligence/Machine Learning (AI/ML)-Based Software as a Medical Device (SaMD) 4 (April 2019), available at US FDA Artificial Intelligence and Machine Learning Discussion Paper.
FDCA § 520(o)(1)(A-E).
See FDCA § 201(h)(1)(B).
See Appendix B, Example 11 of FDA’s Policy for Device Software Functions and Mobile Medical Applications.