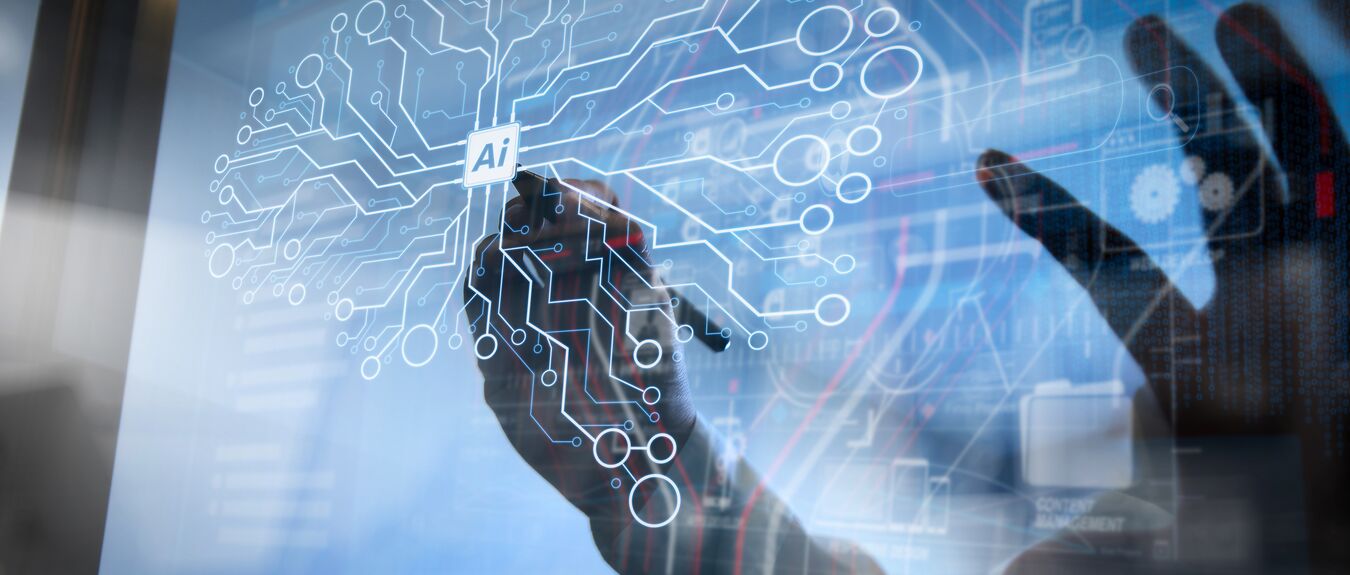
2024-2025 Global AI Trends Guide
Amid continued rapid development of novel software products that present new opportunities in the health care space, FDA has yet to grant marketing authorization to a medical device incorporating artificial intelligence (AI) that continuously learns in the field to create derived audiovisual content, otherwise known as generative AI (GenAI). However, the technology industry does not stand still, and manufacturers are eager to find ways to introduce continually learning algorithms to the medical device space in the U.S. Recognizing the simultaneous existence of significant risks and notable potential associated with GenAI, FDA dedicated the inaugural meeting of its Digital Health Advisory Committee on November 20-21, 2024, to discussing Total Product Lifecycle (TPLC) considerations for GenAI-enabled devices. Consistent with the TPLC approach emphasized by the agency, the meeting was structured in three parts to address first, pre-market considerations associated with GenAI-enabled devices, then risk management, and finally post-market considerations.
In his opening statement, FDA Commissioner Dr. Robert Califf stressed the need to address “disparities rooted in race, ethnicity, education, and geography” that are leading the United States to fall behind other countries in terms of health outcomes, and asserted that AI – on the important condition that it be developed and maintained responsibly – could revolutionize health care delivery, access, and quality. The Director of FDA’s Center for Devices and Radiological Health (CDRH), Dr. Michelle Tarver, echoed the sentiment that there is enormous unmet clinical need and health inequity in this country that could be closed, at least in part, with the help of GenAI.
Unique challenges
Unique characteristics associated with GenAI technology introduce uncertainty around the application and applicability of existing regulatory frameworks for providing oversight and necessary guardrails during product development, market entry, and post-market surveillance. Such characteristics were addressed by the Committee and presenters throughout the discussion, with highlights noted below:
Questions addressed
Much of the DHAC committee discussion was centered on addressing three key questions, summarized below:
Premarket Performance Evaluation
The committee emphasized several critical aspects necessary for evaluating Gen AI-enabled devices in premarket submissions. It was noted that clarifying specific indications for use as well as target populations and usage settings will be crucial for developing products in preparation for premarket performance evaluation. This development process should include careful consideration of limitations of the proposed use, including explicitly reporting cases that may introduce uncertainty, providing demographic information for the intended population, and specifying where devices will be used (e.g., decentralized health care settings). FDA will also expect to understand whether the GenAI is dynamic (adaptive) or static, whether the algorithms operate autonomously or require human intervention (human-in-the-loop vs. human-out-of-the-loop), and details about the modeling and training of the device. Performance review of GenAI devices will direct particular focus to the following requirements:
The Committee discussed potential evaluation strategies for addressing these requirements, including benchmarking performance data with standardized datasets, including expert evaluation for clinical relevance, and model-based evaluation using other models with human oversight to evaluate the subject GenAI device. Data sheets were also explored as a potential tool that may be used to standardize information presented to the agency. Much of the discussion also concentrated on performance from the perspective of the anticipated user of the GenAI device – e.g., how to enable users to make better informed clinical decisions and how to mitigate risks associated with user interfaces.
While GenAI presents a host of unique risks, some themes that emerged from Committee discussion echoed the agency’s thoughts on approaches to regulating AI/ML technologies more broadly. In line with the general emphasis on TPLC, the Committee suggested that post-marketing plans should be included in pre-marketing discussions. There was also a focus on how risks to patient harm underscore governance needs, and the Committee stressed the shared responsibility among manufacturers, physicians, and health systems deploying Gen AI-enabled devices.
Risk Management
As noted previously, Gen AI presents novel opportunities such as enhanced diagnostic capabilities but also introduces unique risks that require new types of monitoring. For example, the Committee discussed how the need to correct AI-generated content after the creation of an initial draft, particularly for text drafts or interpretation of images, will require the development of new control mechanisms. Auditing will also be a key aspect of risk management, and other potential targets for tracking and monitoring for companies to consider were mentioned as well.
Post-Market Performance Monitoring
The discussion focused on what specific strategies and tools can be implemented to monitor and manage the performance and accuracy of a GenAI-enabled device across multiple sites, ensuring consistency while addressing potential regional biases in data variation compared to the authorized device. Specific strategies discussed included:
Additionally, the Committee discussed the specific capabilities that should be included in post-market performance monitoring, which largely fell into three categories, as summarized below:
The Committee discussed some considerations for leveraging PCCPs for adaptive (GenAI-enabled) algorithms. Establishing boundaries or guardrails within PCCPs to define permissible ranges for automatic updates was one point of interest. It was also noted that monitoring post-market performance over time must ensure maintained or improved device effectiveness while accommodating local adaptations across multiple sites. Additionally, panelists stated that updating labeling when modifications occur is crucial, so users receive timely information about device functionality. Shortly after the Committee meeting, FDA issued its final guidance on PCCPs for AI-enabled device software functions; see our discussion of this guidance here.
Conclusion
DHAC's discussions highlighted both the opportunities and challenges of integrating GenAI into health care. While these technologies have transformative potential — enhancing diagnostics and treatment options — the associated risks necessitate careful consideration from industry and regulators alike, and there are still many questions to be answered. Continued dialogue will help shape effective regulatory strategies that foster innovation while ensuring public trust in emerging technologies within health care settings.
Hogan Lovells has been assisting clients in navigating the FDA regulatory process for AI-enabled devices throughout its evolution over the last decade. If you have questions or would like us to help you evaluate an issue related to AI or GenAI in the context of medical device regulation, please contact one of the authors of this alert or the Hogan Lovells attorney with whom you normally work.
Authored by Kelliann Payne, Suzanne Levy Friedman, and Evelyn Tsisin.