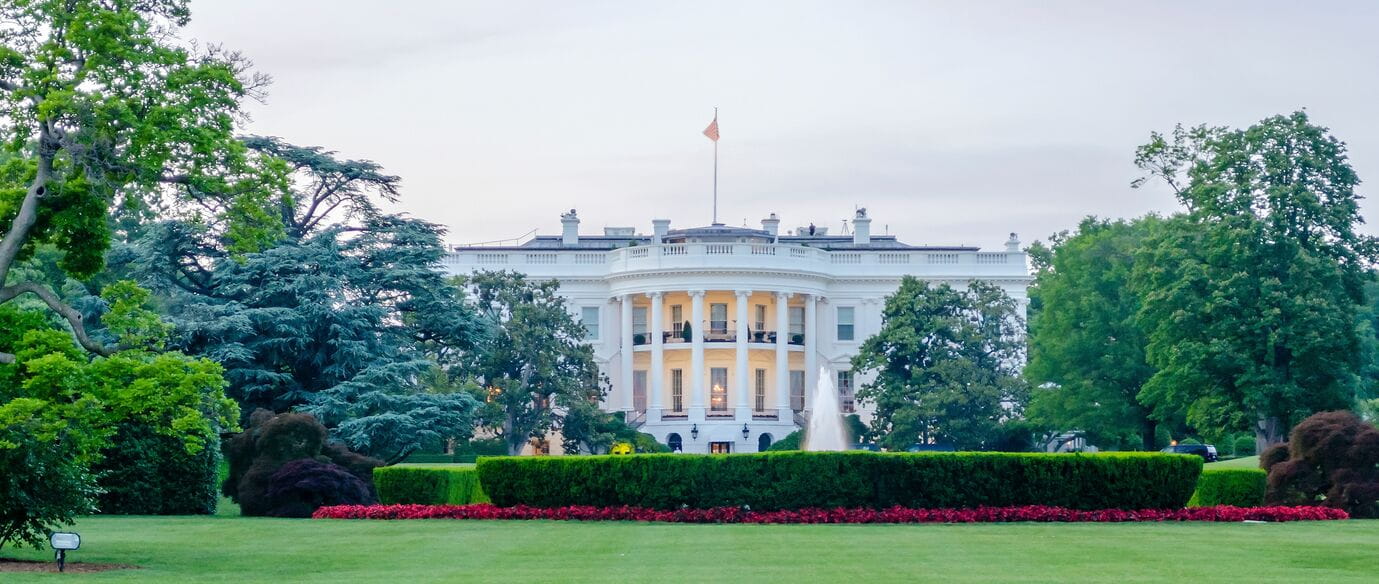
Reflecting on President Trump’s first 100 days in office
On 26 October 2023, the Bank of England (BoE) (including the Prudential Regulation Authority (PRA)), and the Financial Conduct Authority (FCA) (collectively ‘the regulators’) published a feedback statement summarising the responses to the joint discussion paper (DP5/22) on Artificial Intelligence (AI) and machine learning which was published in October 2022. DP5/22 aims to understand how AI may affect the prudential and conduct supervision of financial services firms.
The joint AI and machine learning feedback statement (PRA FS2/23/ FCA FS23/6) provides a summary of the responses to the October 2022 Discussion Paper on AI and machine learning (DP5/22).
The aim of the feedback is to acknowledge and summarise the responses to DP5/22 and identify the main themes emerging from the feedback. It does not include policy proposals but it suggests the regulatory direction of travel and flags how firms are prioritising AI risks and approaching creating AI governance frameworks. DP5/22 received 54 responses from regulated firms, trade bodies, and non-financial businesses. The regulators state that there was no significant divergence of opinion between sectors.
DP5/22 is part of the supervisory authorities’ wider programme of work related to AI, including the AI Public Private Forum (AIPPF), and its final report published in February 2022.
Chapter 2 of DP5/22 outlined the regulators’ objectives and remits, and their relevance to the use of AI in financial services. It surveyed existing approaches by regulators and authorities to distinguishing between AI and non-AI. Some approaches provide a legal definition of AI (for example as seen in the proposed EU AI Act), whereas other approaches have instead identified the key characteristics of AI (for example the UK AI White Paper). DP5/22 asked respondents whether a financial services sector-specific regulatory definition would be beneficial and whether there are other effective approaches that do not rely on a definition.
Most respondents thought that a regulatory definition of AI would not be useful for the following reasons: (i) a definition could become quickly outdated due to the pace of technology development, (ii) definitions could be too broad (i.e. cover non-AI systems) or too narrow (i.e. would fail to cover all the use cases), (iii) a definition could create incentives for regulatory arbitrage, and (iv) creating a sectoral regulatory definition could be in conflict with the regulatory authorities’ technology-neutral approach. Those in the minority that were in favour of a definition suggested that it would help prevent the inconsistent interpretation or implementation. Many respondents pointed to the use of alternative, principles-based or risk-based approaches to the definition of AI with a focus on specific characteristics of AI or risks posed or amplified by AI.
Most respondents suggested that a technology-neutral, outcomes-based, and principles-based approach would be effective in supporting the safe and responsible adoption of AI in financial services. The regulatory focus should be on the outcomes affecting consumers and markets rather than on specific technologies. This outcome-focused approach is in line with the approach of existing regulation, namely, that firms should ensure good outcomes and effective oversight whether or not AI is used in the process. In particular, one respondent suggested that indicators of better outcomes for customers could include the factors already set out by the Consumer Duty. The approach to AI should be proportionate to the risks associated with, or materiality of each specific AI application.
Some respondents welcomed further guidance on the interpretation and evaluation of good consumer outcomes in the AI context with respect to existing sectoral regulations such as the FCA’s Consumer Duty. Guidance on preventing, evaluating, and mitigating bias, with case studies to help illustrate best practice would also be welcomed. Guidance on the use of personal data in AI in the financial services context, supported by case studies to demonstrate what good looks like was also suggested by respondents.
There are a wide range of benefits of AI in financial services for example driving better consumer outcomes, more personalised advice, lower costs, and better prices. DP5/22 also invited responses on potential risks and risk mitigation strategies including those set out below.
A majority of respondents cited consumer protection as an area for the supervisory authorities to prioritise. Respondents said that AI could create risks such as bias, discrimination, lack of explainability, transparency, and exploiting vulnerable consumers or consumers with protected characteristics.
Commenting on market integrity and financial stability, respondents highlighted that the speed and scale of AI could increase the potential for (new forms of) systemic risks, such as interconnectivity between AI systems and the potential for AI-induced firm failures. Respondents mentioned the following potential risks to financial markets: (i) emergence of new forms of market manipulation, (ii) the use of deepfakes for misinformation potentially destabilising financial markets, (iii) third-party AI models resulting in convergent models including digital collusion or herding, and (iv) AI could amplify flash crashes or automated market disruptions.
On governance, while most respondents said that existing firm governance structures are either already sufficient to cover AI or are being adapted by firms to make them sufficient there are concerns that there will be still be risks related to insufficient oversight. Some respondents noted that there may not be sufficient skills and experience within firms to support the level of oversight required to ensure technical (for example data and model risks) and non-technical (for example consumer and market outcomes) risk management. Some respondents noted that a lack of technical expertise is especially worrying given the increased adoption of third-party AI software. Some respondents also pointed out the importance of human-in-the-loop for mitigating risks associated with overreliance on AI or overconfidence in the accuracy of AI.
Respondents suggested that third-party providers of AI solutions should provide evidence supporting the responsible development, independent validation, and ongoing governance of their AI products, providing firms with sufficient information to make their own risk assessment. Respondents argued that third party providers do not always provide sufficient information to enable effective governance of some of their products. Given the scope and ubiquity of third-party AI applications, respondents commented that the risks posed by third party exposure could lead to an increase in systemic risks. Some respondents said that not all firms have the necessary expertise to conduct adequate due diligence of third-party AI applications and models.
Respondents suggested that as the technology develops, there may also be increased access of AI tools by bad actors who wish to use AI for fraud and money laundering. For example, respondents noted that generative AI can easily be exploited to create deepfakes as a way to commit fraud. The technology may make such fraud more sophisticated, greater in scale and harder to detect. This may in turn create risks to consumers and, if sufficient in magnitude, financial stability.
Some respondents noted that the adoption of Generative AI (GenAI) may increase rapidly in financial services. Respondents noted that the risks associated with the use of GenAI are not fully understood, especially risks related to bias, accuracy, reliability, and explainability. Due to ‘hallucinations’ in GenAI outputs, respondents also suggested that there may be risks to firms and consumers relying on or trusting GenAI as a source of financial advice or information.
Respondents remarked that, while existing regulation is sufficient to cover risks associated with AI, there are areas where clarificatory guidance on the application of existing regulation is needed (such as accountability of different parties in outsourcing) and areas of novel risk that may require further guidance in the future. Some respondents suggested that guidance on best practices for responsible AI development and deployment would help firms ensure that they are adopting AI in a safe and responsible manner. AI capabilities change rapidly. Regulators could respond by designing and maintaining ‘live’ regulatory guidance for example periodically updated guidance and examples of best practice. Specific areas of law and regulation that might be adapted to address AI are summarised below.
A number of respondents stressed the relevance and importance to AI of the existing regulatory framework relating to operational resilience and outsourcing, including the PRA’s supervisory statements (SS) 1/21 – Operational resilience: Impact tolerances for important business services and SS2/21 – Outsourcing and third party risk management, as well as the FCA’s PS21/3 – Building operational resilience. Respondents also noted the relevance of the Bank, the PRA and the FCA’s DP3/22 – Operational resilience: Critical third parties to the UK financial sector.
Most respondents did not think that creating a new Prescribed Responsibility (PR) for AI to be allocated to a Senior Management Function (SMF) would be helpful for enhancing effective governance of AI. Most respondents thought that further guidance on how to interpret the ‘reasonable steps’ element of the SM&CR in an AI context would be helpful, although only if it was practical or actionable guidance.
Some respondents noted legal and regulatory developments in other jurisdictions (including the proposed EU AI Act), and argued that international regulatory harmonisation would be beneficial, where possible, particularly for multinational firms. One respondent noted that the development of adequate and flexible cooperation mechanisms supporting information-sharing (or lessons learnt) across jurisdictions could also minimise barriers and facilitate beneficial innovation.
Respondents highlighted legal requirements and guidance relating to data protection. One respondent noted that the way the UK General Data Protection Regulation (UK GDPR) interacts with AI might mean that automated decision making could potentially be prohibited. One response noted regulatory guidance indicating that the 'right to erasure' under the UK GDPR extends to personal data used to train AI models, which could prove challenging in practice given the limited extent to which developers are able to separate and remove training data from a trained AI model. Other respondents argue that, although it is generally recognised that data protection laws apply to the use of AI, there may be a lack of understanding by suppliers, developers, and users, leading to those actors potentially gaming or ignoring the rules. Most respondents argued that there are areas of data regulation that are not sufficient to identify, manage, monitor, and control the risks associated with AI models. Some pointed to insufficient regulation on the topics of data access, data protection, and data privacy (for example to monitor bias). Some respondents thought that regulation in relation to data quality, data management, and operations are insufficient.
Several respondents sought clarification on what bias and fairness could mean in the context of AI models, more specifically, they asked how firms should interpret the Equality Act 2010 and the FCA Consumer Duty in this context. Other respondents asked for more clarity on how data protection / privacy rights interact with AI techniques.
Open banking was suggested as a way of improving data access within financial services and thus facilitate innovation with AI and competition. Lack of access to high-quality data may be a barrier to entry for firms’ adoption of AI. Open banking may help create a more level playing field by providing firms with larger and more diverse datasets, and therefore enabling more effective competition.
Many respondents emphasised the importance of cross-sectoral and cross-jurisdictional coordination as AI is a cross-cutting technology extending across sectoral boundaries. As a consequence, respondents encouraged authorities to ensure coherence and consistency in regulatory approaches across sectoral regulators, such as aligning key principles, metrics, and interpretation of key concepts. Some respondents suggested that the supervisory authorities work with other regulators to reduce and/or prevent regulatory overlaps and clarify the role of sectoral regulations and legislation.
As set out in the responses to DP5/22, since many regulated firms operate in multiple jurisdictions, an internationally coordinated and harmonised regulatory response on AI is critical in ensuring that UK regulation does not put UK firms and markets at a disadvantage. Minimising fragmentation and operational complexity will therefore be key. The supervisory authorities should support collaboration between financial services firms, regulators, academia, and technology practitioners with the aim of promoting competition. Respondents also noted that encouraging firms to collaborate in the development and deployment of AI, such as sharing knowledge and resources, could help reduce costs and improve the quality of AI systems for financial services. Ongoing industry engagement will clearly be important as the regulatory framework for AI continues to develop. We will be closely monitoring developments so please do get in touch with our financial services regulatory and technology specialists listed on the right hand side with any questions.
Authored by Melanie Johnson, Diana Suciu and Alex Nicol.