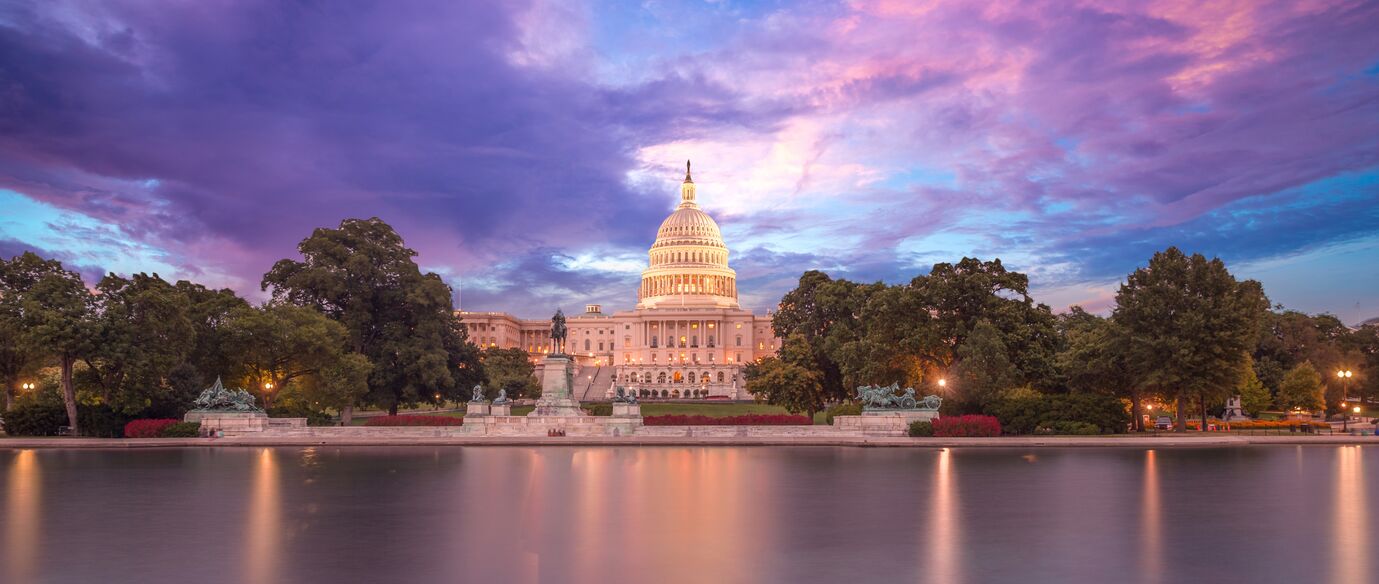
Trump Administration Executive Order (EO) Tracker
New and old attack vectors analyzed by RAND in their report on securing AI weights from theft.
A new report published by RAND highlights the importance of securing the learnable parameters, or weights, of AI models to protect against evolving threats from attackers. The report identifies 38 distinct attack vectors divided into nine categories that can be used by attackers to reach, gain access to, and exfiltrate model weights, their weight values, and the training data underpinning the AI model.
Running Unauthorized Code. Attack vectors within this category include exploiting vulnerabilities for which a patch exists and exploiting individual zero-days.
Compromising Existing Credentials. Attack vectors within this category include traditional forms of social engineering, password brute-forcing and cracking, and expanding illegitimate access.
Undermining the Access Control System Itself. Attack vectors within this category include code vulnerabilities, exploitation of intentional backdoors in algorithms, unauthorized access via encryption vulnerabilities, and access to secret material undermining a protocol.
Bypassing Primary Security System Altogether. Attack vectors within this category include incorrect configuration or security policy implementation that leads to unintentional access and additional copies of weights that may be made vulnerable due to the lack of monitoring and proper oversight.
Nontrivial Access to Data or Networks. Attack vectors within this category include nontrivial access to data networks that can penetrate the environment containing the weights and training data and side-channel attacks that can be used to undermine the security of the model weight by exfiltrating the key that enables authentication to receive access to the weights.
Unauthorized Physical Access to Systems. Attack vectors within this category include direct physical access to sensitive systems and servers containing model weights through various access points such as electronic waste disposal, and malicious placement of portable devices that can manipulate or steal model weights.
Supply Chain Attacks. Attack vectors within this category include gaining unauthorized access through equipment the organization uses, compromising third-party developed code that is incorporated into an organization’s codebase, and targeting vendor access to critical systems.
Human Intelligence. Attack vectors within this category include extortion, bribes, and insider threats.
AI-Specific Attack Vectors. Attack vectors within this category include vulnerabilities in the machine learning stack, the intentional compromise of the machine learning supply chain, prompt-triggered code execution, and model extraction & distillation.
Companies employing and developing AI might consider consulting the report to gain a greater understanding of the threats—and security mitigations—available to them.
Authored by Nathan Salminen and Pat Bruny.
Summer associate Samantha Slack contributed to this article.