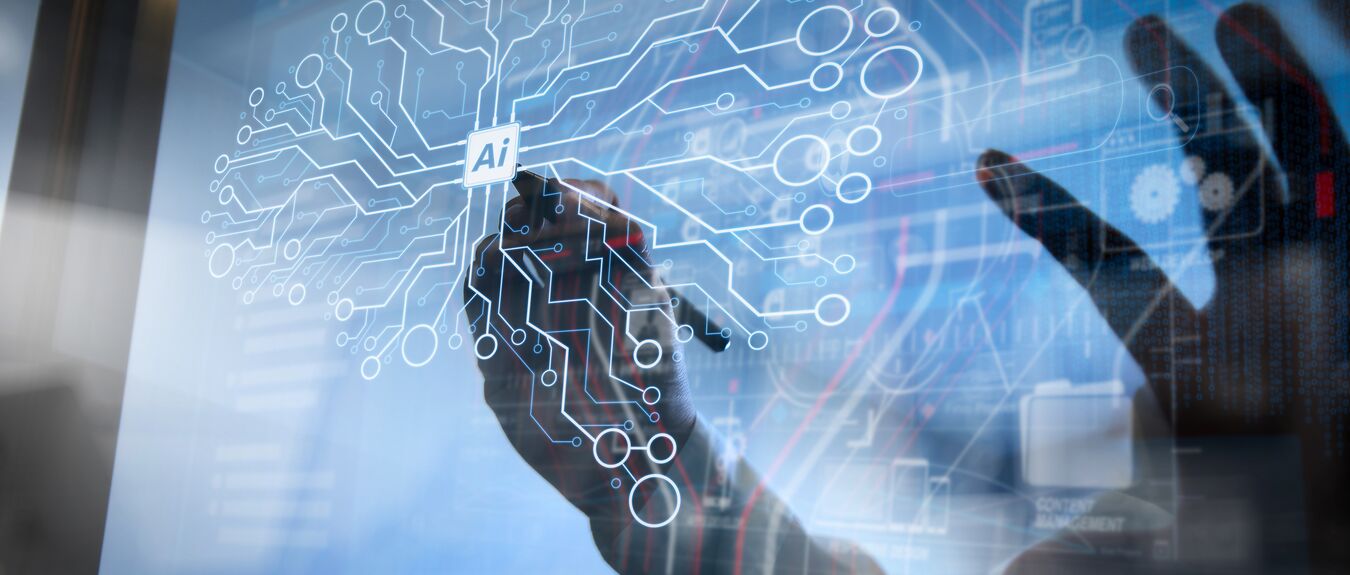
2024-2025 Global AI Trends Guide
Model inversion and membership inference attacks create unique risks to organizations that are allowing artificial intelligences to be trained using their data. Companies may wish to begin to evaluate ways to mitigate risks of these attacks as they train and develop generative AI systems or engage with third party vendors that use their data to train models.
As companies endeavour to incorporate AI into their businesses and engage with vendors that seek to use their customers’ data to train models, it is important to understand the risks associated with the models being adopted or developed. This article highlights two distinct, increasingly significant threats that companies may wish to keep in mind: model inversion and membership inference.
Model inversion is a type of attack that uses the final output of an AI model to identify the original dataset on which the AI system was trained. In some cases, these attacks can allow attackers to reconstruct the information (including sensitive characteristics) that was used to train the model. Essentially, the attacker uses one artificial intelligence to query another artificial intelligence, and asks the malicious artificial intelligence to determine what training data the victim artificial intelligence would have needed to be trained on in order to respond as it does.
For an overly simplified example, imagine that a threat actor suspected that a particular artificial intelligence was trained using non-public profile data from a social media platform, and the threat actor would like to know what states each of the users’ profiles indicated they reside in. Perhaps the malicious artificial intelligence might ask the target artificial intelligence what vegetable people from Idaho like most, and if the artificial intelligence says “potatoes,” it could then iterate through each user’s name asking if that person is likely to enjoy potatoes. The answers might then hint at whether that user lives in Idaho. Then perhaps it asks what sports team the user is likely to support, and so forth, until, after asking dozens of questions about that user related to their state, it might be able to develop a high level of confidence about where the model thinks they live. After asking billions of questions more clever than that, the malicious artificial intelligence may be able to recreate some of the data that the model was trained on.
These risks are especially prevalent when a model is “overfitting,” in which the model essentially remembers certain pieces of training data and generates outputs which closely or identically resemble the training data, rather than generating a unique synthesis of that data.
Two examples of model inversion attacks are Typical Instance Reconstruction Attacks (TIR Attacks) and Model Inversion Attribute Inference Attacks (MIAI Attacks). In a TIR Attack, an adversary could take an image or visual media generated by an AI model and use it to assemble near-accurate images of the individual(s) featured in the training data. Alternatively, in a MIAI Attack, adversaries use information they already have about certain individuals to uncover specific sensitive attributes about them within a model’s training data, which could enable access to information like medical records and financial information.
Membership inference involves exploiting the training processes of machine learning models to identify whether specific individual pieces of data were included in training datasets. For example, when a person is part of the training data, the model tends to show higher confidence in predictions about them, indicating their inclusion.
A useful hypothetical example posed by the UK’s Information Commissioner’s Office illustrates this kind of attack in the context of a hospital. Using membership inference, attackers could use a predictive model based on hospital records alongside other available personal information to infer if an individual had visited a particular hospital during the data collection period. Though this would not reveal the individual’s actual data included in the training set, it would nonetheless show that the individual had an association with the hospital and had sought care there within a certain window.
Model inversion and membership inference each pose many substantial risks:
While the risks posed by model inversion and membership inference may appear daunting, there are many practical steps companies can take to protect against these vulnerabilities.
Artificial intelligence is developing at a rapid pace, and its continued positive advancements naturally bring developments in potential threats and vulnerabilities as well. Model inversion and membership inference are but a few of these new and emerging threats, and companies may wish to continually evaluate their AI governance programs and how they engage with third parties to ensure that they are prepared to meet these challenges.